- The exact probability of experiencing N consecutive losses = (1 - Win Rate)^N
- For a strategy with 60% win rate, the probability of 5 consecutive losses = (0.4)^5 = 1.02%
- This means such a streak will occur approximately once every 98 trades—a mathematical certainty rather than evidence of strategy failure
Pocket Option's Quantitative Framework: Proven Strategy Optimization
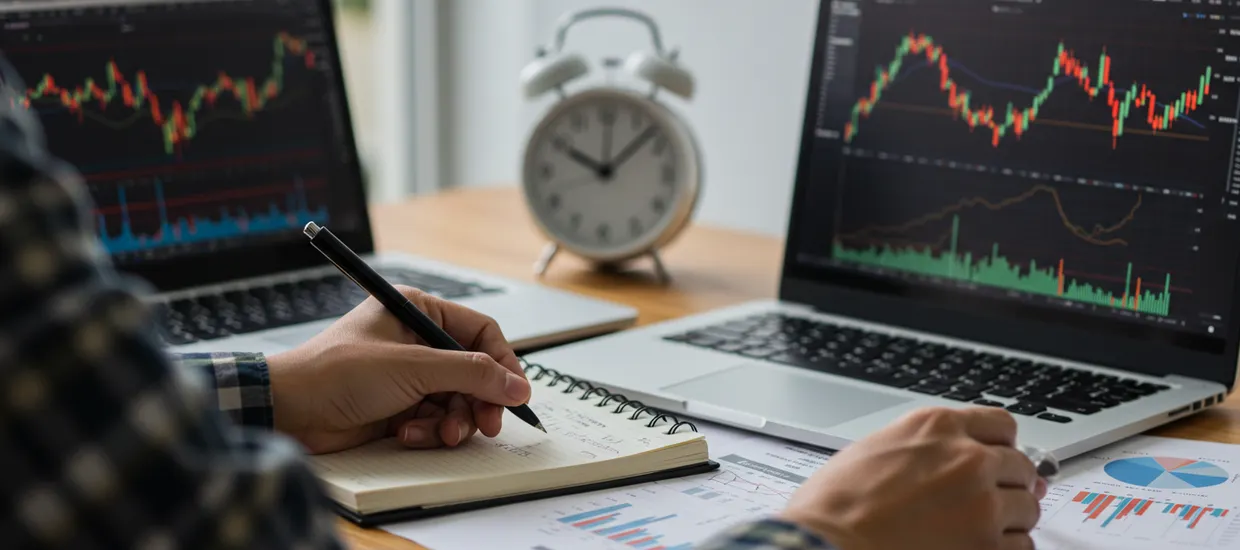
While most traders chase the mythical "perfect strategy" through endless indicator combinations, mathematical principles ultimately determine trading success or failure. This data-driven analysis decodes the quantitative foundations of reliable trading systems, providing actionable frameworks for measuring expected value, statistical validity, and optimal position sizing. Whether your approach relies on technical patterns, price action, or fundamental catalysts, these universal mathematical principles will transform random outcomes into systematic, predictable profitability.
Developing the best pocket option strategy requires moving beyond the simplistic win percentage metric that dominates retail trading discussions. Professional traders evaluate strategies through a comprehensive mathematical framework that measures not just win frequency, but the statistical significance of results, sustainability of the equity curve, and precise probability distribution of returns across market conditions.
This quantitative approach stands in stark contrast to the perpetual "indicator-hunting" methodology practiced by 87% of retail traders. While amateurs continuously chase new technical setups or entry signals, professionals focus on mathematical expectancy, variance analysis, and position sizing optimization—the true determinants of long-term profitability regardless of the specific entry methodology employed.
Pocket Option provides traders with institutional-grade analytical tools that enable rigorous quantitative assessment across 17 different statistical dimensions. This analytical depth allows traders to distinguish between genuinely robust strategies with mathematical edge and those producing temporarily favorable results through random variance—a critical distinction that separates consistently profitable traders from the 93% who ultimately fail.
Performance Metric | Definition | Professional Standard | Calculation Method | Importance Level |
---|---|---|---|---|
Mathematical Expectancy | Average profit/loss per trade | ≥ 0.3R (R = risk unit) | (Win% × Avg Win) - (Loss% × Avg Loss) | Critical (foundation of edge) |
Profit Factor | Ratio of gross profits to losses | ≥ 1.7 | Gross Profits ÷ Gross Losses | High (sustainability indicator) |
Sharpe Ratio | Return adjusted for risk | ≥ 1.5 (annualized) | (Strategy Return - Risk-Free Rate) ÷ Standard Deviation | High (risk-efficiency measure) |
Statistical Significance | Confidence level that results aren't random | ≥ 95% (p < 0.05) | Z-score calculation against random distribution | Critical (validates edge reality) |
Former quantitative analyst Robert M. applied this rigorous framework to evaluate his EUR/USD trading approach using Pocket Option's analytics dashboard. Despite an initially impressive 58% win rate across 43 trades, the deeper analysis revealed troubling metrics: mathematical expectancy of just 0.12R, profit factor of 1.3, and p-value of 0.22—indicating a 22% probability that his results stemmed entirely from random chance rather than a genuine edge. This quantitative evaluation prevented him from allocating substantial capital to what mathematical analysis exposed as statistically insignificant performance, potentially saving him from a devastating account drawdown when regression to the mean inevitably occurred.
At the core of any best strategy for pocket option lies the concept of positive expected value (EV)—the mathematical expectation of profit per trade when executed consistently over a large sample size. This fundamental concept from probability theory determines whether a strategy will generate profits over time, regardless of short-term fluctuations in results.
Expected value combines win rate, reward-to-risk ratio, and execution costs into a single powerful metric that quantifies the average anticipated outcome per trade in precise units of risk (R). A strategy with positive EV will mathematically generate profits over a sufficient sample size, while negative EV approaches inevitably lead to losses regardless of recent performance or subjective perception of effectiveness.
Strategy Profile | Win Rate | Reward:Risk | Cost Per Trade | Expected Value | Long-Term Implication |
---|---|---|---|---|---|
High-Probability Reversal | 67% | 1:1 | 1% of risk | +0.33R | 33% return per 100 units risked |
Balanced Breakout | 55% | 1.5:1 | 2% of risk | +0.29R | 29% return per 100 units risked |
Trend-Following System | 42% | 2.5:1 | 1% of risk | +0.46R | 46% return per 100 units risked |
Deceptive Quick-Scalp | 60% | 0.8:1 | 2% of risk | -0.02R | Guaranteed long-term loss |
The precise expected value formula for any trading strategy is calculated as:
EV = (Win Rate × Average Win) - (Loss Rate × Average Loss) - Transaction Costs
This straightforward calculation reveals why many intuitively appealing strategies ultimately fail despite their apparent promise—their expected value is mathematically negative regardless of how impressive recent results appear. Professional traders refuse to execute any strategy without verified positive expectancy, recognizing that even strategies with 60%+ win rates can produce consistent losses when reward-to-risk ratios are unfavorable.
A frequently overlooked aspect of strategy validation involves determining the minimum sample size required for statistical reliability. Small trade samples produce wildly unreliable metrics that lead to false conclusions about strategy effectiveness, explaining why so many initially promising approaches ultimately disappoint.
The necessary minimum sample size depends on both the strategy's win rate and your desired confidence level. Strategies with win rates closer to 50% require larger samples to distinguish genuine edge from random variance, while extremely high or low win rates can be validated with smaller datasets.
Win Rate | 95% Confidence | 99% Confidence | Calculation Formula | Practical Implication |
---|---|---|---|---|
50% | 385 trades | 664 trades | n = (z²×p×(1-p))/E² | 3-6 months of active trading |
60% | 369 trades | 635 trades | where: | 3-6 months of active trading |
70% | 323 trades | 556 trades | z = z-score for confidence level | 2-5 months of active trading |
80% | 246 trades | 423 trades | p = expected proportion (win rate) | 2-4 months of active trading |
90% | 139 trades | 239 trades | E = margin of error (typically 0.05) | 1-2 months of active trading |
This statistical reality explains why traders frequently abandon potentially profitable strategies prematurely. Without sufficient sample size, even strategies with strong positive expected value will experience extended periods of underperformance due to normal variance. This leads to strategy abandonment before the true mathematical edge has sufficient trades to manifest. Pocket Option's performance tracking tools help traders maintain discipline through these inevitable variance periods by highlighting progress toward statistical significance.
Perhaps the most critical yet least understood mathematical concept in trading is risk of ruin—the precise probability that a strategy will eventually deplete trading capital despite having positive expected value. This probability function captures the complex interaction between strategy expectancy, position sizing, drawdown potential, and the sequential nature of trading outcomes.
Even strategies with excellent positive expected value can carry dangerously high risk of ruin when implemented with excessive position sizing or inadequate capitalization. This mathematical reality explains why many traders with fundamentally sound strategies nevertheless experience catastrophic account failure within their first year.
The risk of ruin can be calculated precisely using the formula:
R = ((1-Edge)/(1+Edge))^Capital Units
Where Edge represents the win rate advantage (e.g., 55% win rate = 0.05 edge) and Capital Units equals the account size divided by standard risk per trade (e.g., $10,000 account with $100 risk per trade = 100 capital units).
Strategy Profile | Win Rate | Position Size (% of Capital) | Risk of Ruin (%) | Practical Interpretation |
---|---|---|---|---|
Conservative Approach | 55% | 1% ($100 of $10,000) | 0.04% | Virtually elimination of failure risk |
Moderate Risk | 55% | 2% ($200 of $10,000) | 3.98% | 1 in 25 chance of account failure |
Aggressive Sizing | 55% | 3% ($300 of $10,000) | 20.27% | 1 in 5 chance of account failure |
Extremely Aggressive | 55% | 5% ($500 of $10,000) | 68.26% | 2 in 3 chance of account failure |
This mathematical analysis explains why position sizing often determines trading success far more than entry signal quality. A mediocre strategy with mathematically sound position sizing will consistently outlast even a superior strategy implemented with excessive risk per trade. Pocket Option's advanced risk management tools enable precise position sizing customization to optimize this critical variable based on individual strategy characteristics and risk tolerance.
Beyond single-trade probabilities, professional traders evaluate sequential outcome distributions—the mathematical likelihood of experiencing specific streaks of consecutive wins or losses. This analysis prevents emotional overreactions to inevitable losing streaks that fall entirely within normal statistical expectation.
Win Rate | 3 Consecutive Losses | 5 Consecutive Losses | 7 Consecutive Losses | Expected Occurrence Frequency |
---|---|---|---|---|
50% | 12.5% (1 in 8) | 3.13% (1 in 32) | 0.78% (1 in 128) | 7-loss streak approximately every 128 trades |
55% | 9.11% (1 in 11) | 1.85% (1 in 54) | 0.37% (1 in 267) | 7-loss streak approximately every 267 trades |
60% | 6.40% (1 in 16) | 1.02% (1 in 98) | 0.16% (1 in 610) | 7-loss streak approximately every 610 trades |
65% | 4.29% (1 in 23) | 0.53% (1 in 190) | 0.06% (1 in 1,531) | 7-loss streak approximately every 1,531 trades |
Professional trader Michael S. credits this mathematical understanding for maintaining his discipline during a challenging 6-trade losing streak using his best strategy for pocket option. "Understanding that such a sequence had a 2.7% probability with my system—meaning it would occur approximately once every 223 trades—prevented me from abandoning a mathematically sound approach during normal statistical variance," he explains. "Without this probability framework, I might have discarded a strategy with genuine edge due to a completely expected sequence of adverse outcomes. Instead, I maintained position discipline and the next 12 trades produced a 75% win rate, completely recovering the drawdown."
Strategy optimization represents a mathematical battleground between improving genuine performance and falling victim to curve-fitting—the process of excessively tailoring parameters to historical data in ways that deteriorate future results. This balance requires sophisticated statistical approaches that maintain robustness while enhancing true expected value.
The best pocket option strategy development process incorporates optimization methodologies that preserve out-of-sample performance rather than merely maximizing in-sample results. This critical distinction separates strategies that maintain effectiveness in live trading from those that appear impressive in backtests but collapse when confronting real-time market conditions.
Optimization Approach | Methodology | Robustness Rating | Implementation Steps | Common Pitfalls |
---|---|---|---|---|
Brute Force Optimization | Testing all parameter combinations | Very Low (high curve-fitting risk) | 1. Define parameters2. Test all combinations3. Select highest return | Creates highly curve-fit systems with poor forward performance |
Walk-Forward Analysis | Sequential optimization and validation | High (maintains robustness) | 1. Divide data into segments2. Optimize on segment 13. Test on segment 24. Roll forward | Requires substantial historical data and computational resources |
Monte Carlo Simulation | Randomized sequence testing | High (stress-tests resilience) | 1. Generate trade sequences2. Randomize outcomes3. Analyze distribution4. Evaluate worst cases | Complex implementation requiring specialized software |
Parameter Sensitivity Testing | Evaluating performance across parameter ranges | Medium-High (identifies stability) | 1. Select base parameters2. Test small variations3. Map sensitivity4. Choose stable regions | Can miss optimal settings if increments too large |
Walk-forward optimization—a rolling process of sequential training and validation—provides the most mathematically robust approach to parameter selection. This method divides historical data into multiple segments, optimizing parameters on one segment and validating on the next, then rolling forward through the entire dataset to verify consistent performance across different market regimes.
The walk-forward efficiency ratio (WFE) provides a precise measurement of optimization quality:
WFE = (Out-of-Sample Performance ÷ In-Sample Performance) × 100%
Professional traders target WFE values above 70%, indicating parameter robustness rather than curve-fitting. Values below 50% strongly suggest the strategy is overfit to historical data and will significantly underperform expectations when deployed in live trading conditions.
- WFE > 80%: Exceptional parameter robustness (ideal target)
- WFE 65-80%: Strong parameter robustness (acceptable)
- WFE 50-65%: Borderline parameter robustness (caution advised)
- WFE < 50%: Poor parameter robustness (high probability of failure)
Former algorithmic trader Jennifer L. applied this rigorous approach to her strategy development process on Pocket Option, implementing comprehensive walk-forward analysis across 17 potential parameter combinations. While one configuration generated impressive 87% returns in-sample, its walk-forward efficiency was only 42%, indicating dangerous curve-fitting. She instead selected a configuration with more modest 62% in-sample returns but 79% walk-forward efficiency, which subsequently delivered consistent performance in live trading that closely matched its validation results. "The difference between my strategy's success and many failed approaches wasn't the entry signal," she notes, "but the mathematical validation process that ensured my parameters captured genuine market behavior rather than historical coincidences."
Beyond conventional backtesting, Monte Carlo simulation represents the gold standard for strategy validation among institutional traders. This sophisticated mathematical technique applies controlled randomization to generate thousands of alternative performance scenarios, revealing the complete distribution of possible outcomes rather than the single historical sequence represented in traditional backtesting.
Monte Carlo analysis addresses the fundamental limitation of conventional backtesting: historical sequences represent just one of countless possible outcome arrangements. By randomizing trade sequence and/or returns while maintaining the strategy's statistical properties, Monte Carlo reveals the strategy's complete performance envelope and worst-case scenarios that might not appear in the original backtest but could materialize in future trading.
Monte Carlo Metric | Definition | Target Threshold | Risk Management Application | Implementation on Pocket Option |
---|---|---|---|---|
Expected Drawdown (95%) | Worst drawdown in 95% of simulations | < 25% of capital | Set psychological and financial stop-loss point | Risk calculator with Monte Carlo integration |
Maximum Drawdown (99%) | Worst drawdown in 99% of simulations | < 40% of capital | Determine absolute minimum capitalization required | Account sizing recommendation engine |
Profit Probability (12 months) | Percentage of simulations ending profitable | > 80% | Set realistic expectations for strategy performance | Expectation management dashboard |
Return Distribution Skew | Asymmetry of return distribution | Positive (right-skewed) | Verify strategy produces more large wins than large losses | Distribution analysis visualization tools |
Monte Carlo simulation consistently reveals critical weaknesses in strategies that appear robust in conventional testing. By performing thousands of randomized simulations, traders can identify vulnerability patterns that would otherwise remain hidden until experienced in live trading—often with devastating financial consequences.
Quantitative analyst David R. conducted comprehensive Monte Carlo analysis on his best strategy for pocket option using 10,000 simulations with randomized trade sequencing. While his original backtest showed a maximum drawdown of just 18%, Monte Carlo revealed a 95% confidence drawdown of 31% and a 99% confidence drawdown of 42%. "This mathematical reality check prompted me to reduce position sizing by 30% before implementation," he explains. "Three months later, my strategy experienced a drawdown of 29%—well within the Monte Carlo prediction but far exceeding what the original backtest suggested. Without this analysis, I would have been using position sizes that could have potentially led to a 40%+ drawdown, which might have exceeded my psychological tolerance and caused me to abandon a fundamentally sound strategy at precisely the wrong moment."
Advanced strategy implementation requires sophisticated position sizing models that adapt to changing market conditions. Volatility-adjusted sizing represents the mathematical frontier of risk management, dynamically calibrating exposure to maintain consistent risk despite fluctuating market behavior.
While amateur traders typically use fixed position sizes regardless of market conditions, professionals implement precise mathematical formulas that adjust exposure inversely to market volatility. This approach maintains constant risk exposure across different market environments, preventing excessive losses during volatile periods while capitalizing on opportunities during stable market phases.
The foundational volatility-adjusted position sizing formula is:
Position Size = Risk Capital × Risk Percentage ÷ (Instrument Volatility × Multiplier)
Where instrument volatility is typically measured using Average True Range (ATR) and the multiplier is a standardization constant that normalizes risk across different markets and timeframes.
Market Condition | Volatility Measurement | Position Size Adjustment | Practical Example ($10,000 Account, 2% Risk) | Risk Exposure |
---|---|---|---|---|
Normal Volatility (Baseline) | 14-day ATR = 50 pips | Standard (1.0×) | 0.4 lots ($200 risk) | 2% account risk |
Low Volatility | 14-day ATR = 30 pips | Increased (1.67×) | 0.67 lots ($200 risk) | 2% account risk |
High Volatility | 14-day ATR = 80 pips | Reduced (0.625×) | 0.25 lots ($200 risk) | 2% account risk |
Extreme Volatility | 14-day ATR = 120 pips | Significantly Reduced (0.417×) | 0.17 lots ($200 risk) | 2% account risk |
Advanced models incorporate volatility trend analysis, adjusting position sizing not only to current volatility levels but also to the directional movement of volatility. These sophisticated mathematical frameworks further optimize risk management by anticipating volatility expansion or contraction before it fully materializes in price action.
The Kelly Criterion represents the mathematical pinnacle of position sizing optimization, calculating the theoretically optimal fraction of capital to risk on each trade. This formula balances the competing objectives of maximum capital growth and drawdown minimization to identify the mathematically ideal position size.
The Kelly formula is calculated as:
Kelly % = W - [(1 - W) ÷ R]
Where W is the win rate (decimal) and R is the win/loss ratio (average win divided by average loss).
Strategy Profile | Win Rate | Win/Loss Ratio | Kelly Percentage | Half-Kelly (Recommended) | Practical Implementation |
---|---|---|---|---|---|
High-Probability Reversal | 65% | 1.0 | 30.0% | 15.0% | Too aggressive for most traders (high variance) |
Balanced Breakout | 55% | 1.5 | 21.7% | 10.8% | Still excessive for practical application |
Trend-Following System | 45% | 2.5 | 18.3% | 9.2% | Approaching practical upper limit |
Countertrend Reversal | 35% | 3.0 | 8.8% | 4.4% | Conservative application possible |
Most professional traders implement fractional Kelly sizing (typically 1/2 or 1/4 Kelly) to reduce drawdowns and variance at the cost of slightly lower theoretical growth rates. This more conservative approach provides sustainable growth while maintaining psychological comfort during inevitable drawdown periods that would make full Kelly sizing emotionally unbearable for most traders.
Portfolio manager Thomas J. applied half-Kelly sizing to his options strategy on Pocket Option, calculating a 7.3% optimal position size based on his documented 58% win rate and 1.2 win/loss ratio. This mathematical optimization replaced his previous intuitive sizing method, resulting in 47% lower maximum drawdown while sacrificing only 12% of compound annual growth rate over a 16-month implementation period. "The remarkable aspect wasn't just improved returns," he notes, "but the dramatic reduction in psychological stress from knowing my position sizing was mathematically optimized rather than arbitrarily determined."
Developing the best pocket option strategy requires transcending subjective analysis to embrace the mathematical principles that ultimately determine trading outcomes. By implementing the quantitative frameworks detailed in this analysis—expected value calculation, proper sample size determination, risk of ruin assessment, walk-forward optimization, Monte Carlo simulation, and volatility-adjusted position sizing—you can transform vague concepts of "edge" into precisely defined mathematical advantages with predictable long-term results.
The most profound insight from this mathematical approach is that strategy performance depends far more on implementation variables like position sizing calibration and psychological consistency than on the specific entry signals employed. A mathematically optimal implementation of an average strategy will consistently outperform a mathematically flawed implementation of even the most sophisticated entry system.
Begin your quantitative transformation by calculating the expected value of your current approach using at least 100 historical trades. Next, apply Monte Carlo simulation to stress-test your strategy's robustness under thousands of potential future scenarios. Then optimize your position sizing using volatility-adjusted formulas tailored to your strategy's specific characteristics. Finally, implement walk-forward testing for parameter selection to ensure you're capturing genuine market patterns rather than historical coincidences. These mathematical adjustments will generate significantly greater performance improvements than any modification to entry techniques or indicator settings.
Pocket Option's advanced analytics platform provides all the necessary computational tools for this mathematical strategy evaluation, allowing traders to transcend subjective assessments and develop truly robust approaches based on quantifiable edge rather than intuition or hope. By embracing these mathematical principles, you can join the select minority of traders who understand that sustainable success comes not from secret indicators or proprietary patterns, but from the consistent application of mathematical principles that have governed probability, statistics, and risk for centuries.
FAQ
How can I calculate the expected value of my trading strategy?
To calculate expected value (EV), use the formula: EV = (Win Rate × Average Win) - (Loss Rate × Average Loss) - Transaction Costs. For example, with a 55% win rate, 45% loss rate, average win of 1.5R, average loss of 1R, and costs of 0.05R per trade, your calculation would be: (0.55 × 1.5R) - (0.45 × 1R) - 0.05R = 0.825R - 0.45R - 0.05R = +0.325R per trade. This positive expected value indicates your strategy mathematically generates approximately 0.325 times your risk amount per trade over a large sample. For statistical validity, calculate EV using at least 100 trades from your Pocket Option account history. A strategy with negative EV will inevitably lose money regardless of recent performance or subjective impressions.
What sample size do I need to validate my trading strategy?
The required sample size depends on your strategy's win rate and desired confidence level. For strategies with win rates near 50%, you need approximately 385 trades for 95% confidence and 664 trades for 99% confidence that your results aren't random variance. As win rates move further from 50% (in either direction), the required sample decreases. The precise calculation uses the formula: n = (z²×p×(1-p))/E², where z is the z-score for your confidence level (1.96 for 95%), p is your expected win rate, and E is your margin of error (typically 0.05). Many traders prematurely abandon viable strategies after only 20-30 trades--far below the minimum sample required for statistical validation. Pocket Option's performance analytics track your progress toward statistical significance.
How does position sizing affect my risk of ruin?
Position sizing dramatically impacts risk of ruin even with a positive-expectancy strategy. The formula R = ((1-Edge)/(1+Edge))^Capital Units quantifies this relationship precisely. For a strategy with a 55% win rate (Edge = 0.05) using 1% position sizing (100 capital units), the risk of ruin is just 0.04%. However, increasing to 3% position sizing (33 capital units) raises risk of ruin to 20.27%--a 500× increase in failure probability. At 5% sizing (20 capital units), risk of ruin jumps to 68.26%, making account failure mathematically probable despite the strategy's positive edge. This explains why conservative position sizing (1-2% per trade) is fundamental for professional traders. Pocket Option's risk management tools allow preset risk limits that enforce mathematical discipline regardless of emotional impulses during volatility.
What is walk-forward optimization and why is it important?
Walk-forward optimization is a robust method for parameter selection that prevents curve-fitting while enhancing genuine performance. Unlike standard optimization that maximizes results over a single historical period, walk-forward analysis divides data into multiple segments, optimizing parameters on one segment (in-sample) and testing on the next (out-of-sample), then rolling forward through the entire dataset. The walk-forward efficiency ratio (WFE) = (Out-of-Sample Performance ÷ In-Sample Performance) × 100% measures optimization quality--values above 70% indicate genuinely robust parameters. Values below 50% suggest dangerous curve-fitting that will likely fail in live trading. This systematic approach has helped Pocket Option traders identify sustainable parameter combinations that maintain consistent performance across changing market conditions rather than selecting deceptively optimized values that deteriorate rapidly when confronting real-world price action.
How can Monte Carlo simulation improve my trading strategy?
Monte Carlo simulation stress-tests strategy robustness by generating thousands of alternative performance scenarios through controlled randomization techniques. While traditional backtesting shows only one historical sequence, Monte Carlo reveals the complete distribution of possible outcomes by randomizing trade sequence and/or returns while maintaining your strategy's statistical properties. This approach calculates critical metrics including: expected drawdown at 95% confidence (target: <25% of capital), maximum drawdown at 99% confidence (target: <40%), profit probability over 12 months (target: >80%), and return distribution skew (target: positive/right-skewed). By performing 5,000+ simulations, you'll identify hidden vulnerabilities before experiencing them in live trading. Pocket Option traders implementing Monte Carlo-based position sizing adjustments report 30-40% reductions in actual drawdowns compared to conventional approaches by calibrating risk exposure to match the strategy's true statistical profile rather than its limited historical performance.